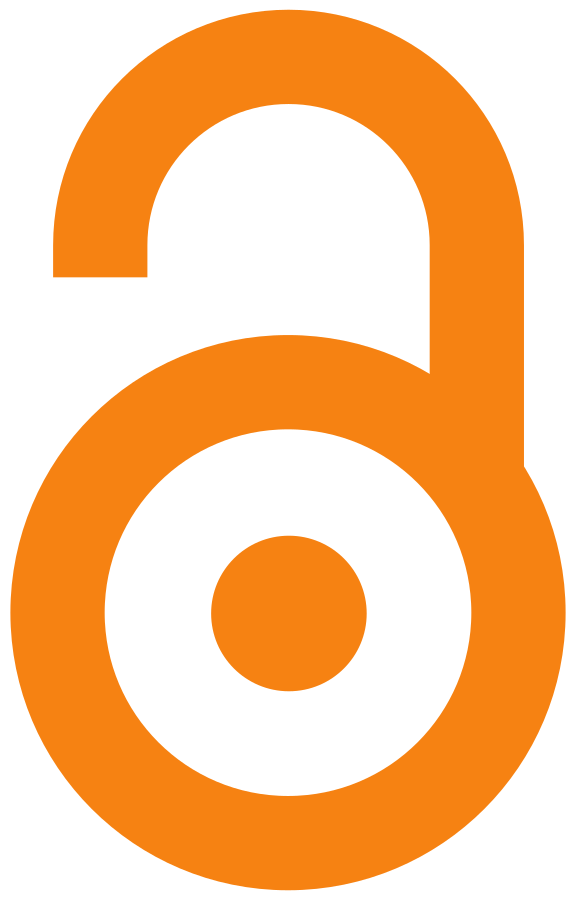
EVALUATION OF TRANSPORT FLOW USING DEEP LEARNING METHODS OF SATELLITE IMAGES
Abstract
The exponential growth of urbanization has led to increasing problems in traffic management, which require innovative solutions for efficient estimation of traffic flow. Deep learning methods are emerging as a powerful tool for processing and analyzing satellite images, and are being used for traffic flow estimation. This paper describes deep learning-based methods for traffic flow estimation using satellite imagery.
Keywords
Transport Flow, satellite images, urban traffic
References
Antoniou, C.; Barcelo, J.; Brackstone, M.; Celikoglu, H.B.; Ciuffo, B.; Punzo, V.; Sykes, P.; Toledo, T.; Vortisch, P.; Wagner, P. Traffic Simulation: Case for Guidelines. 2014. Available online: https://data.europa.eu/doi/10.2788/11382 (accessed on 8 April 2019).
Salehi, B.; Zhang, Y.; Zhong, M. Automatic moving vehicles information extraction from single-pass worldView-2 imagery. IEEE J. Sel. Top. Appl. Earth Obs. Remote Sens. 2012, 5, 135–145. [Google Scholar] [CrossRef]
Satellite Imaging Corporation. IKONOS Satellite Sensor. 2017a. Available online: https://www.satimagingcorp.com/satellite-sensors/ikonos/ (accessed on 18 March 2020).
Satellite Imaging Corporation. QuickBird Satellite Sensor. 2017b. Available online: https://www.satimagingcorp.com/satellite-sensors/quickbird/ (accessed on 18 March 2020).
Mace, E.; Manville, K.; Barbu-McInnis, M.; Laielli, M.; Klaric, M.; Dooley, S. Overhead Detection: Beyond 8-bits and RGB. arXiv 2018, arXiv:1808.02443. [Google Scholar]
Zhihuan, W.; Xiangning, C.; Yongming, G.; Yuntao, L. Rapid target detection in high resolution remote sensing images using YOLO Model. Int. Arch. Photogramm. Remote Sens. Spat. Inf. Sci.-ISPRS Arch. 2018, 42, 1915–1920. [Google Scholar]
Article Statistics
Downloads
Copyright License
Copyright (c) 2024 Hamrakulov Botirbek

This work is licensed under a Creative Commons Attribution 4.0 International License.