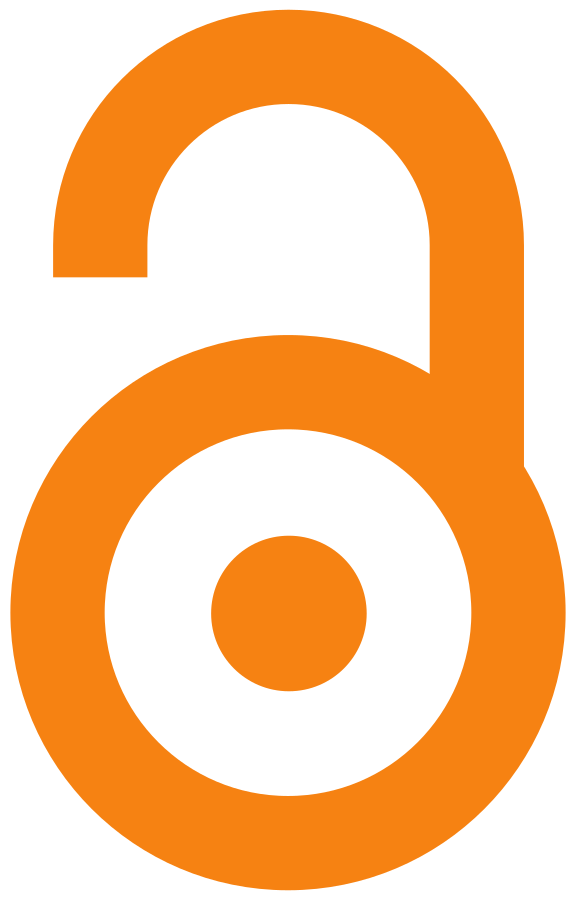
HARNESSING MACHINE LEARNING FOR ACCURATE CROP AND YIELD PREDICTION
Abstract
Accurate crop and yield prediction is crucial for optimizing agricultural productivity, managing food security, and supporting sustainable farming practices. This study presents a machine learning-based approach to predict crop yields by analyzing various environmental, soil, and weather-related factors. Using data from agricultural regions, the model incorporates variables such as rainfall, temperature, soil properties, and crop type to enhance the accuracy of yield predictions. Several machine learning algorithms, including decision trees, random forests, and neural networks, are evaluated for performance, with a focus on predictive accuracy, computational efficiency, and scalability. The results demonstrate that machine learning can significantly improve the precision of crop yield forecasts compared to traditional statistical methods. This model has the potential to assist farmers, policymakers, and agricultural businesses in making informed decisions related to crop management, resource allocation, and market planning. Ultimately, the study highlights the transformative role of machine learning in advancing precision agriculture and ensuring sustainable agricultural growth.
Keywords
Machine Learning, Crop Prediction, Yield Forecasting
References
M.Ananthara, T. Arunkumar, and R. Hemavathy, Cry: An improved crop yield prediction model using bee hive clustering approach for agricultural data sets,” in Pattern Recognition, Informatics and Medical Engineering (PRIME), 2013 IEEE International Conference , pp. 473- 478.
U.P. Narkhede and K.P.Adhiya, A Study of Clustering Techniques for Crop Prediction - A Survey, American International Journal of Research in Science, Technology, Engineering Mathematics, vol 1, Issue 5, ISSN no: 2328- 3491, pp. 45-48 ,2014.
Department of agriculture, Maharashtra state, Accessed on12-Feb-2014.[On-line].Available: http://www.mahaagri.gov.in/.
D.M. Kiri L. Wagstaff and and S. R. Sain, Harvist: A system for agricultural and weather studies using advanced statistical methods,” 2005. [Online]. Available: https://www.agriskmanagementforum.org.
R.A.A. and K. R.V., Review - role of data mining in agriculture,” International Journal of Computer Science and Information Technologies(IJCSIT), 2013,vol. 4(2),no. 0975- 9646, pp. 270-272.
M.Kannan, S.Prabhakaran, and P.Ramachandran, Rainfall forecasting using data mining technique,” International Journal of Engineering and Technology, vol. 2, no.0975- 4024, pp. 397-401, 2010.
S.Kim and Wilbur, “ An EM clustering algorithm which produces a dual representation," Proceedings of 10th IEEE International Conference on Machine Learning and Applications and Workshops (ICMLA), vol. 2, pp. 90- 95,2011.
Article Statistics
Downloads
Copyright License
Copyright (c) 2024 Shreya P. Bhanose

This work is licensed under a Creative Commons Attribution 4.0 International License.